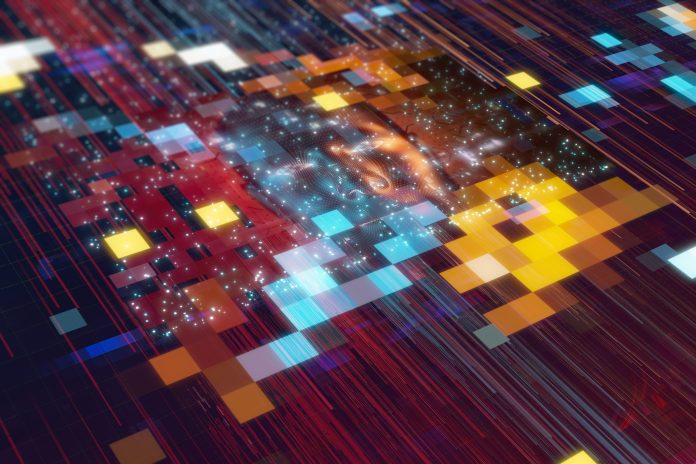
Dr Hassan Khan, Head of School of Digital Finance at Arden University, describes the growing influence of AI in the financial industry
Late last year, the Financial Conduct Authority (FCA) and the Bank of England (BoE) analysed the impact of artificial intelligence AI in the financial industry in the UK. Looking at machine learning (ML), in particular, they found that the number of UK financial services firms using ML is rising, with 72% reporting that they either use or develop ML applications. These applications are being used in more and more areas of business, largely due to the rapid development of such technology. As such, ML is now embedded into many day-to-day operations.
AI in the financial industry is used frequently, with the sector having the highest number of ML applications. This is likely due to its most commonly identified benefits: enhanced data and analytics capabilities, increased operational efficiency and improved fraud and money laundering detection. The financial industries least likely to increase their adoption of ML are non-bank lenders and capital and investment markets. Despite this, the banking and financial services industry is set to become the largest investor in AI in 2023.
The pros of adopting AI in the financial industry
Adopting AI in the financial industry has many benefits. One of the most significant advantages is its capacity to automate time-consuming jobs, resulting in increased productivity. Because ML algorithms can process large volumes of data, not only will financial institutions become more efficient, but they will also be able to provide more specialised, personalised solutions for customers. Because of this, we expect AI will enable financial institutions to become more competitive and provide better customer service.
Consumers are hungry for financial independence and want ease of access to their funds. Allowing them the chance to do so is the driving force behind adopting AI in personal finance and banking. An early example of AI in personal finance is Capital One’s Eno, which launched the first natural language SMS text-based assistant offered by a U.S. bank. It generates insights and anticipates customer needs, such as alerting customers about suspected fraud or price hikes in subscription services. This was back in 2017, and AI has significantly improved since then.
AI techniques are also used in asset management, buy- side asset allocation, and stock selection market activities. This is made possible through ML models, which can detect signals and understand the underlying relationships in large datasets. Additionally, AI techniques are applied to optimise operational workflows and manage risks.
In traditional algorithmic trading, programmers create a set of if/then rules that govern the trading process. In other words, it still needs human input to determine the factors that control trades. With AI algorithmic trading, ML can handle such transactions independently, as it’s constantly filtering information from what’s happening in the market.
In digitised markets, such as equities and FX markets, AI algorithms can enhance liquidity management and the execution of large orders with minimal market impact, by optimising size, duration and order size dynamically, based on market conditions. Traders can also use AI for risk and order flow management purposes for better efficiency and to streamline implementation. However, some AI software can be expensive and is often reserved for larger asset managers or institutional investors who can invest.
The cons to adopting AI in the financial industry
AI applications in finance can present financial and non- financial risks. There is concern about protecting consumers and investors – most of which pertain to data handling. The volume, ubiquitousness, and continuously flowing nature of data used in AI systems raises questions regarding data protection and privacy concerns. It could also create biases and unfair outcomes. On top of this, there are added cyber security risks and issues that point towards consumers not being fully aware of the risks or how their data is being used due to unclear legal jargon in consent forms that are often skimmed over.
AI in the financial industry could also potentially harm market competition – especially if only the big players can afford the most intelligent AI. Similar to non-AI models, using the same ML models by many finance practitioners could also prompt herding behaviour. This can result in one-way markets, which in turn may raise risks around liquidity and the stability of systems, especially during stressful periods.
On top of this, there is always the risk of AI missing out or not considering unforeseen scenarios or extreme markets – especially due to it being taught on historical events. This may create negative outcomes if there is little to no human interaction in trading, and such outcomes can cause significant disruptions in financial markets and result in unexpected losses.
Lack of transparency in AI model processes
Finally, the lack of transparency in AI model processes could also conflict with existing financial policies and governance frameworks. This could challenge the unbiased approach to policymaking.
We need clear policies regarding AI usage that support AI innovation in line with protecting financial consumers and promoting a fair, transparent financial market.
We expect that guidelines will continue to emphasise human decision-making – especially regarding decisions that directly impact consumers, such as lending.